Transforming Healthcare with AI
Project MONAI is revolutionizing medical imaging through a comprehensive ecosystem of AI tools. Our mission is to bridge the gap between research innovation and clinical implementation, providing enterprise-grade solutions that accelerate the advancement of healthcare technology.
PyTorch Native
Built on PyTorch for seamless integration and flexibility
Research Ready
Advanced tools for medical AI research
Standardized
Best practices for healthcare AI development and research
Community Driven
Supported by global healthcare experts
Innovation Focused
Cutting-edge AI architectures and methods
Open Source Design
Apache 2.0 licensed for maximum flexibility and collaboration
End-to-End Medical AI Lifecycle
From data annotation to clinical deployment, MONAI provides a comprehensive toolkit that ensures quality and consistency at every stage of the medical AI development process.
MONAI Label
Intelligent image annotation powered by AI assistance.
- Active learning for efficient data selection
- Multiple viewer integrations
- AI-Assisted annotation
- Multi-user collaboration support
MONAI Core
Domain-specific framework for training state-of-the-art medical imaging AI models.
- Medical-specific transforms
- UNETR architecture
- Pre-trained model zoo
- Automated ML pipelines
MONAI Deploy
Robust framework for deploying AI models in clinical settings.
- Clinical workflow integration
- DICOM & FHIR support
- Containerized deployment with MAP
- Inference optimization
Community & Support
Join our growing community of researchers, developers, and healthcare professionals.
Success Stories
Discover how leading healthcare institutions and industry partners are implementing MONAI to transform medical imaging workflows.
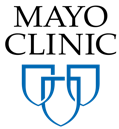
AI Integration in Clinical Imaging
The Center for Augmented Intelligence in Imaging at Mayo Clinic Florida demonstrates seamless integration of MONAI-compatible AI models into clinical Radiology workflows. Their implementation has enhanced operational efficiency, improved decision-making processes, and led to better patient outcomes through streamlined clinical workflows.
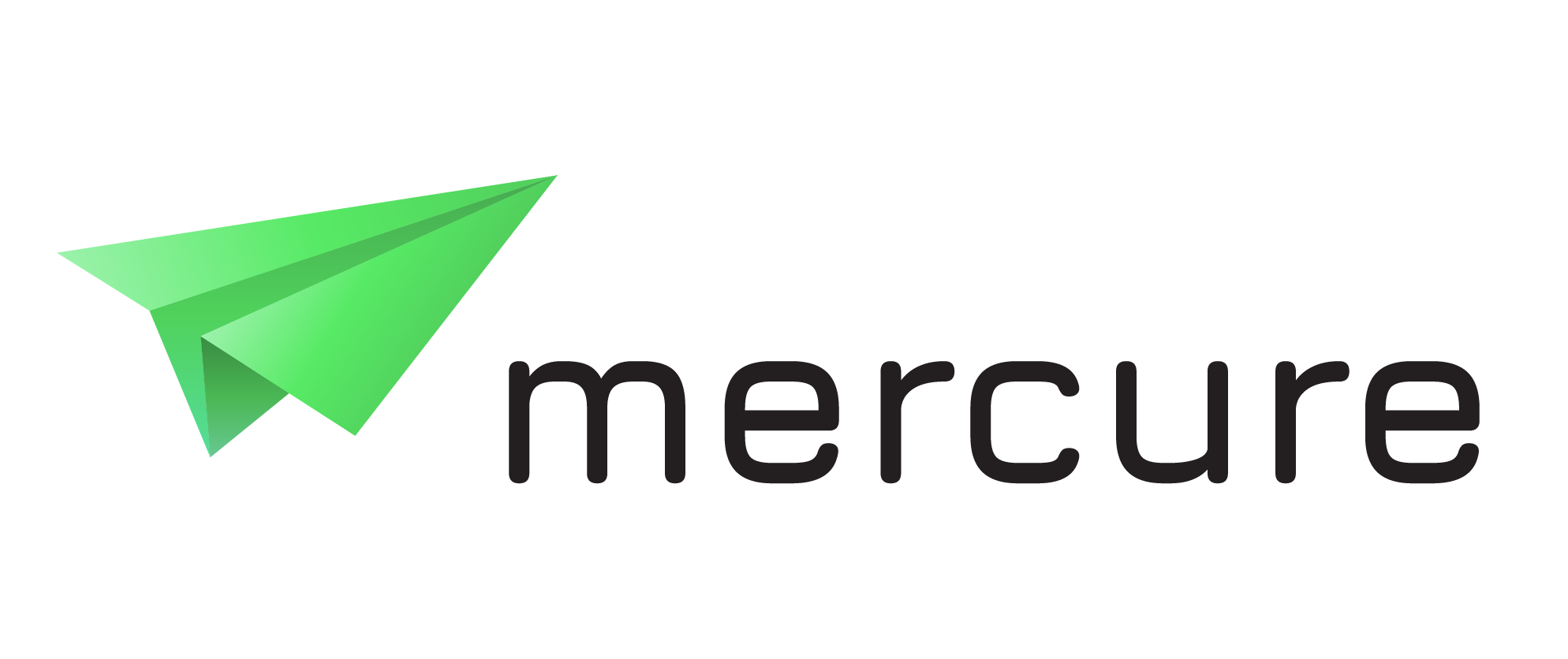
Rapid Deployment with Mercure DICOM
The integration of MONAI Application Packages (MAPs) with the Mercure DICOM Orchestrator has revolutionized AI deployment in clinical environments. This partnership delivers seamless DICOM integration, comprehensive monitoring capabilities, and a flexible routing system that adapts to various clinical workflows.
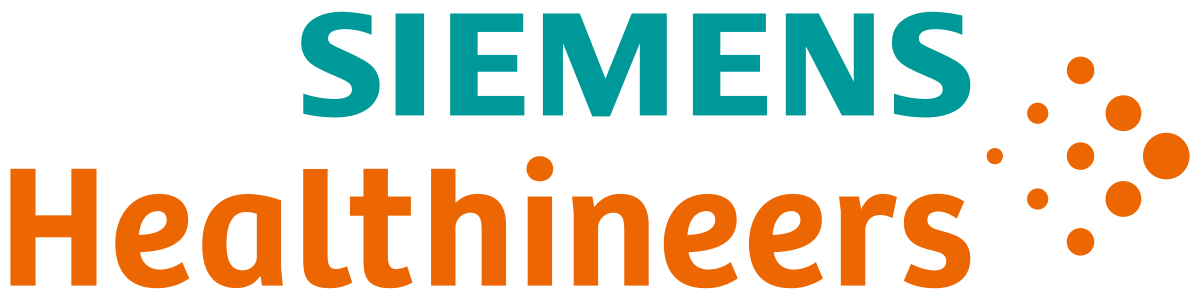
Digital Marketplace Integration
Siemens Healthineers has transformed healthcare delivery by adopting MONAI Deploy for their Digital Marketplace. This integration enables enterprise-scale deployment across their global healthcare network, providing standardized AI deployment solutions that benefit healthcare providers worldwide.
Contributors
Over the last three years our community has expanded rapidly! But it takes a community to build out the success of Project MONAI, which is why we want to highlight contributing organizations. Below, you'll find contributors organizations who have dedicated resources to actively contributing back to Project MONAI.
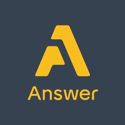
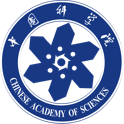
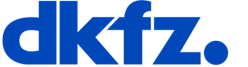
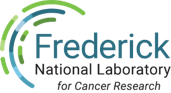
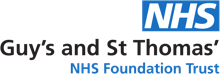
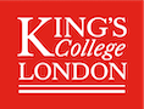
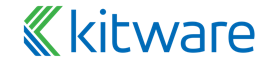
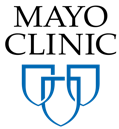
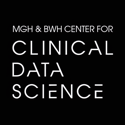
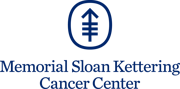
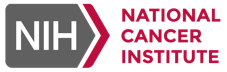
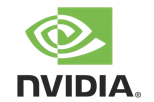
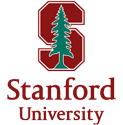
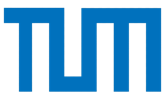
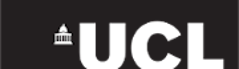
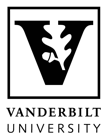
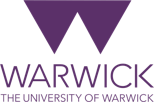